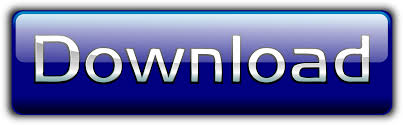
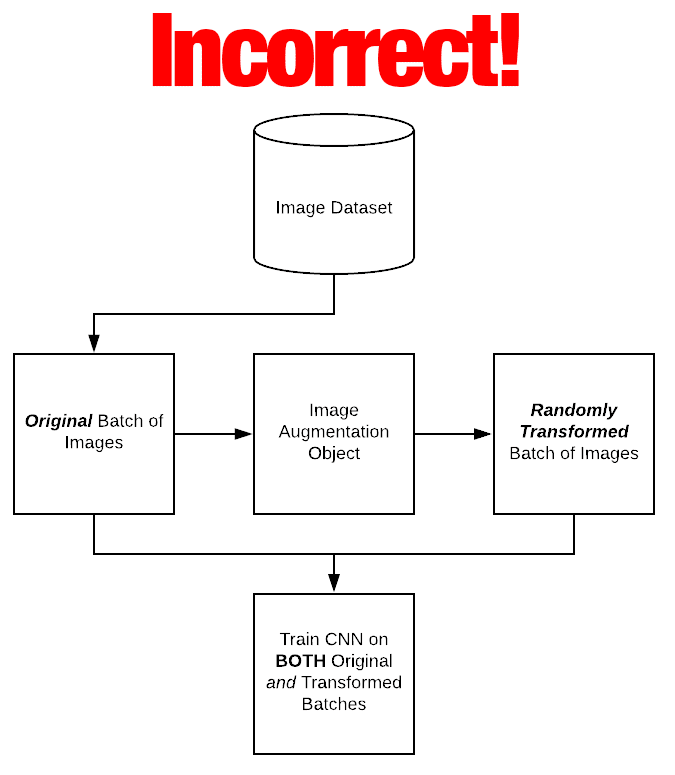
In the real world scenario, we may have a dataset of images taken in a limited set of conditions. This essentially is the premise of data augmentation. More specifically, a CNN can be invariant to translation, viewpoint, size or illumination (Or a combination of the above). Data Augmentation in playĪ convolutional neural network that can robustly classify objects even if its placed in different orientations is said to have the property called invariance. Our neural network would think these are distinct images anyway. Minor changes such as flips or translations or rotations. So, to get more data, we just need to make minor alterations to our existing dataset. For instance, a poorly trained neural network would think that these three tennis balls shown below, are distinct, unique images. Why? Because, neural networks aren’t smart to begin with. You don’t need to hunt for novel new images that can be added to your dataset. How do I get more data, if I don’t have “more data”? Also, the number of parameters you need is proportional to the complexity of the task your model has to perform. Naturally, if you have a lot of parameters, you would need to show your machine learning model a proportional amount of examples, to get good performance. State of the art neural networks typically have parameters in the order of millions! Our optimization goal is to chase that sweet spot where our model’s loss is low, which happens when your parameters are tuned in the right way. When you train a machine learning model, what you’re really doing is tuning its parameters such that it can map a particular input (say, an image) to some output (a label).
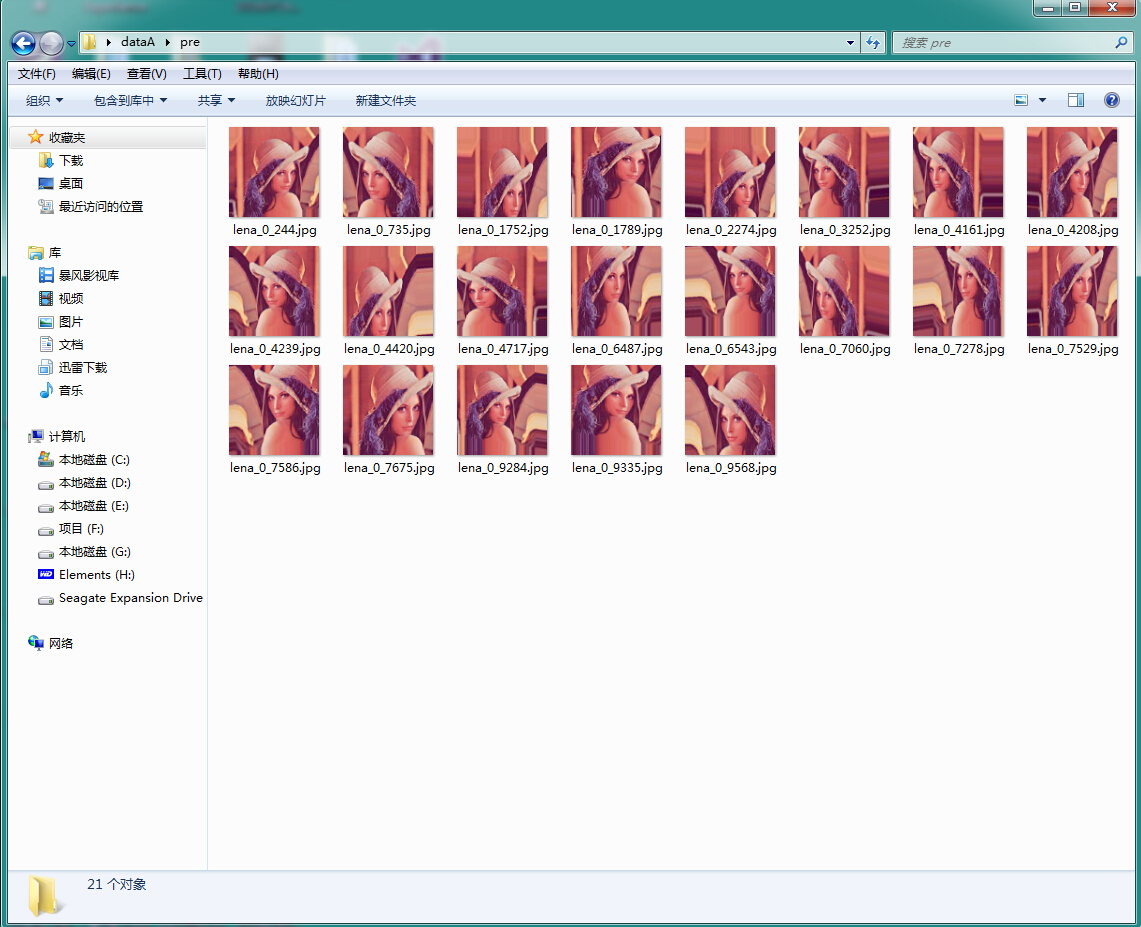
Why is there a need for a large amount of data? Number of parameters (in millions), for popular neural networks. The answer is, yes! But before we get into the magic of making that happen, we need to reflect upon some basic questions. Feeling disappointed, you wonder can my “state-of-the-art” neural network perform well with the meagre amount of data I have? You also recall someone mentioning having a large dataset is crucial for good performance. You recall that most popular datasets have images in the order of tens of thousands (or more). Chances are, you find a dataset that has around a few hundred images. Feeling ebullient, you open your web browser and search for relevant data. You have a stellar concept that can be implemented using a machine learning model.

KERAS DATA AUGMENTATION MNSIT HOW TO
This is Part 2 of How to use Deep Learning when you have Limited Data. model.fit_generator(data_generator.This article is a comprehensive review of Data Augmentation techniques for Deep Learning, specific to images. data_generator.flow_from_directory('path_to_dir')įit_generator function used to train a neural network. Images in the directory are stored in subdirectories with respect to their class. data_generator.flow(X,Y)įlow_from_directory function generates batches of augmented data located at specific directory of a disk.
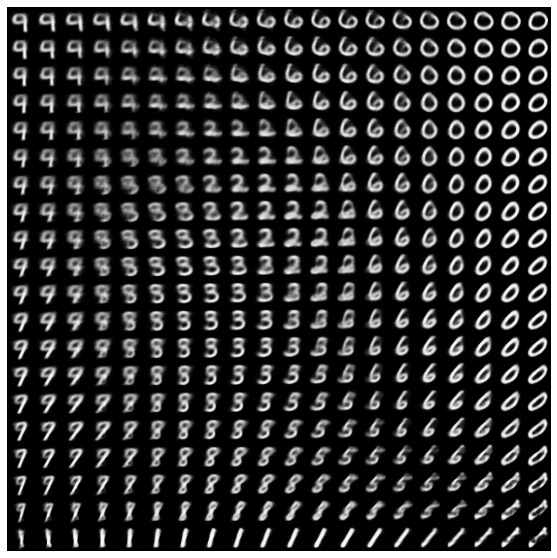
from import ImageDataGeneratorįlow function loads the image dataset in memory and generates batches of augmented data. Here, is the example of how to use ImageDataGenerator class. An ImageDataGenerator class function provide a range of transformations. Image Augmentation using Keras ImageDataGeneratorĪ Keras deep learning library provides the data augmentation function, which applies augmentation automatically while training the model. The numpy package provides a flip operation. Here, I have illustrated various data augmentation technique with example in python.įlip augmentation derived the horizontal flip and vertical flip. It is also important to select the specific data augmentation method carefully in the context of the problem domain. However, we can generate more data with some modifications. But, the small amount of train data is not sufficient to get high performance. In Real-life, we may have limited train data in various condition. Neural networks would not distinguish the augmented image. These transformations include many operations from the field of image manipulations, like flips, rotate at a various angle, shifts, zooms and many more. Training the neural network on more data leads to achieving higher accuracy. Data Augmentation is a technique of creating new data from existing data by applying some transformations.
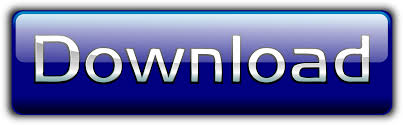